
Suppose there are n observations, where W is a projection operation. Several methods have been proposed to estimate PLMs. On the other hand, PLMs are more flexible than the standard linear models since they combine both parametric and nonparametric components. The PLM is a special form of the additive regression models Hastie and Tibshrani (1990) Stone (1985), which allows easier interpretation of the effect of each variables and may be preferable to a completely nonparametric regression since the well-known reason -gcurse of dimensionality-h. PLMs are defined by Y = X-À + g(T) + -Ã, (5.1) where X and T are d-dimensional and scalar regressors, -À is a vector of unknown parameters, g(-E) an unknown smooth function and -Ã an error term with mean zero conditional on X and T. Numerous examples illustrate the implementation in practice.

When the model is heteroscedastic, the variance functions are approximated by weighted least squares estimators. kernel regression, spline approximation, piecewise polynomial and local polynomial techniques. More specifically, we are mainly concerned with least squares estimators of the linear parameter while the nonparametric part is estimated by e.g. This chapter covers the basic results and explains how PLMs are applied in the biometric practice. PLMs generalize standard linear regression techniques and are special cases of additive models. Partially linear models (PLM) are regression models in which the response depends on some covariates linearly but on other covariates nonparametrically. 34 - 38) like Bioinformatics, Medical Imaging, Finance, Econometrics and Network Intrusion Detection highlight the usefulness of computational statistics in real-world applications. Lastly, a set of selected applications (Chs.
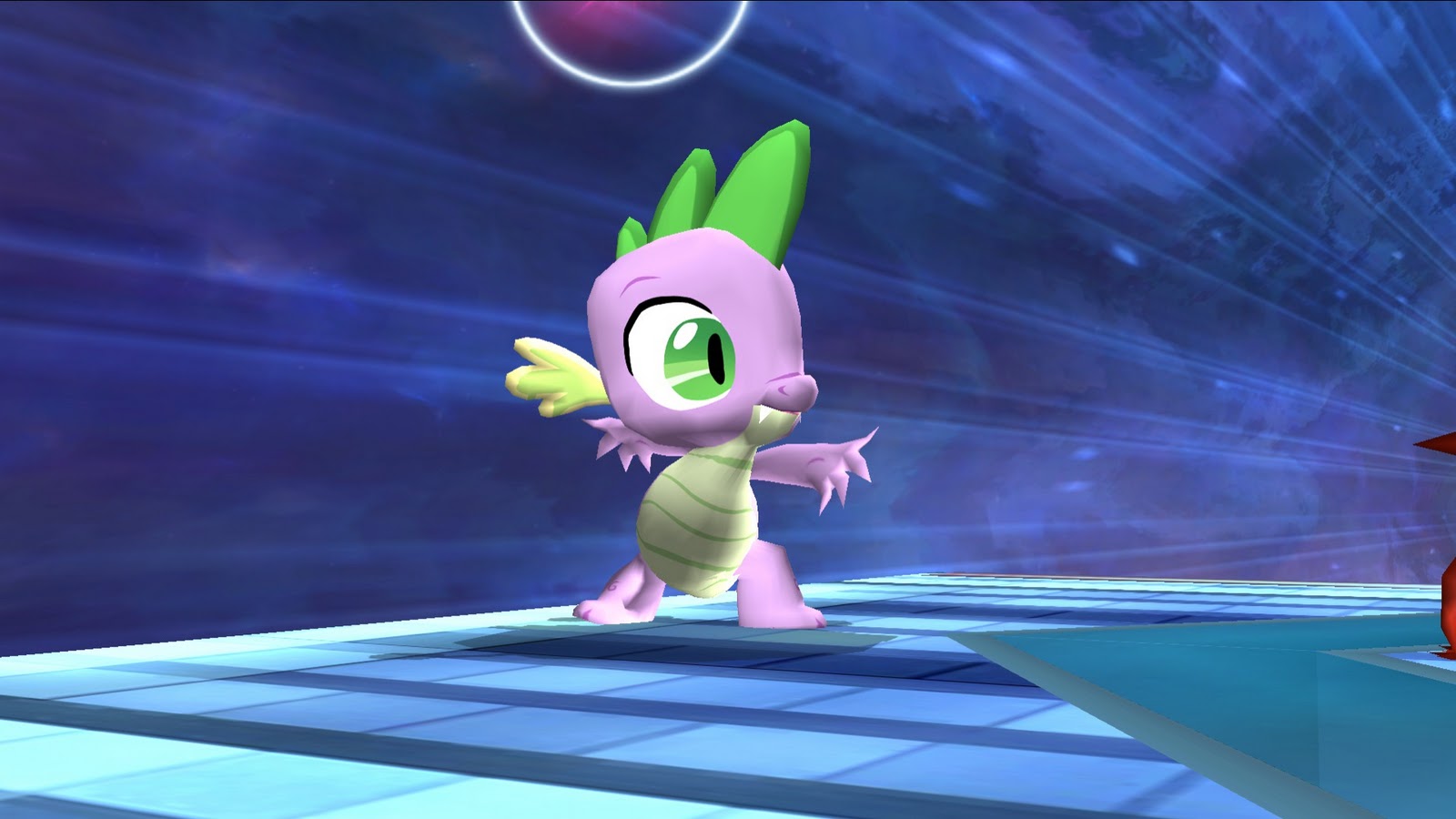
Special attention is given to smoothing, iterative procedures, simulation and visualization of multivariate data. 16 - 33) focuses on statistical methodology. Emphasis is placed on the need for fast and accurate numerical algorithms, and some of the basic methodologies for transformation, database handling, high-dimensional data and graphics treatment are discussed. 2 - 15) presents several topics in the supporting field of statistical computing. It begins with "How Computational Statistics became the backbone of modern data science" (Ch.1): an overview of the field of Computational Statistics, how it emerged as a separate discipline, and how its own development mirrored that of hardware and software, including a discussion of current active research. This new edition is divided into 4 parts in the same way as the first edition. The Handbook of Computational Statistics - Concepts and Methods (second edition) is a revision of the first edition published in 2004, and contains additional comments and updated information on the existing chapters, as well as three new chapters addressing recent work in the field of computational statistics.
